Abstract
Background
Metabolic syndrome (MetS) is a cluster metabolic disorder that includes central obesity, insulin resistance, hypertension, and dyslipidemia, and is highly associated with an increased risk of developing non-communicable diseases (NCDs). This study aimed to compare the reliability of anthro-metabolic indices [visceral adiposity index (VAI), body roundness index (BRI), and a body shape index (BSI), body adiposity index (BAI), lipid accumulation product (LAP), waist to hip ratio, and waist to height ratio] in predicting MetS in Iranian older people.
Methods
This cross-sectional study was conducted based on the data of 2426 adults aged ≥60 years that participated in the second stage of the Bushehr Elderly Health (BEH) program, a population-based prospective cohort study being conducted in Bushehr, Iran. MetS was defined based on the revised National Cholesterol Education Program Adult Treatment Panel III (NCEP-ATP III) criteria. The receiver operating characteristic (ROC) curve analysis was used to assess predictive performance of anthro-metabolic indices and determine optimal cutoff values. Logistic regression analysis was applied to determine the associations between MetS and indices.
Results
2426 subjects (48.1% men) with mean ± SD age of 69.34 ± 6.40 years were included in the study. According to ATP III criteria, 34.8% of men and 65.2% of women had MetS (P < 0.001). Of the seven examined indices, the AUCs of VAI and LAP in both genders were higher than AUCs of other anthro-metabolic indices. Also, in general population, VAI and LAP had the greatest predictive power for MetS with AUC 0.87(0.86–0.89) and 0.87(0.85–0.88), respectively. The lowest AUC in total population belonged to BSI with the area under the curve of 0.60(0.58–0.62). After adjusting for potential confounders (e.g. age, sex, education, physical activity, current smoking) in the logistic regression model, the highest OR in the total population was observed for VAI and LAP, which was 16.63 (13.31–20.79) and 12.56 (10.23–15.43) respectively. The lowest OR for MetS was 1.93(1.61–2.30) for BSI.
Conclusion
This study indicated that both VAI and LAP are the most valuable indices among the anthro-metabolic indices to identify MetS among the elderly in both genders. So, they could be used as proper assessment tools for MetS in clinical practice. However, the cost-benefit of these indices compared to the ATP III criteria need further studies.

Similar content being viewed by others
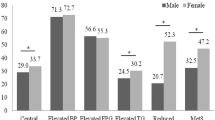
References
Shin D, Kongpakpaisarn K, Bohra C. Trends in the prevalence of metabolic syndrome and its components in the United States 2007–2014. Int J Cardiol. 2018;259:216–9.
Rochlani Y, Pothineni NV, Kovelamudi S, Mehta JL. Metabolic syndrome: pathophysiology, management, and modulation by natural compounds. Ther Adv Cardiovasc Dis. 2017;11(8):215–25.
Khamseh ME, Ansari M, Malek M, Shafiee G, Baradaran H. Effects of a structured self-monitoring of blood glucose method on patient self-management behavior and metabolic outcomes in type 2 diabetes mellitus. J Diabetes Sci Technol. 2011;5(2):388–93.
Mazloomzadeh S, Khazaghi ZR, Mousavinasab N. The prevalence of metabolic syndrome in Iran: a systematic review and meta-analysis. Iran J Public Health. 2018;47(4):473.
Nikbakht H-A, Rezaianzadeh A, Seif M, Ghaem H. Prevalence of metabolic syndrome and its components among a population-based study in south of Iran, PERSIAN Kharameh cohort study. Clin Epidemiol Glob Health. 2020;8(3):678–83.
Gadde KM, Martin CK, Berthoud H-R, Heymsfield SB. Obesity: pathophysiology and management. J Am Coll Cardiol. 2018;71(1):69–84.
Jayawardena R, Ranasinghe P, Ranathunga T, Mathangasinghe Y, Wasalathanththri S, Hills AP. Novel anthropometric parameters to define obesity and obesity-related disease in adults: a systematic review. Nutr Rev. 2020;78(6):498–513.
Prentice AM, Jebb SA. Beyond body mass index. Obes Rev. 2001;2(3):141–7.
Nuttall FQ. Body mass index: obesity, BMI, and health: a critical review. Nutr Today. 2015;50(3):117.
Ryan JM, Crowley VE, Hensey O, McGahey A, Gormley J. Waist circumference provides an indication of numerous cardiometabolic risk factors in adults with cerebral palsy. Arch Phys Med Rehabil. 2014;95(8):1540–6.
Gadekar T, Dudeja P, Basu I, Vashisht S, Mukherji S. Correlation of visceral body fat with waist–hip ratio, waist circumference and body mass index in healthy adults: a cross sectional study. Med J Armed Forces India. 2020;76(1):41–6.
Shao J, Yu L, Shen X, Li D, Wang K. Waist-to-height ratio, an optimal predictor for obesity and metabolic syndrome in Chinese adults. J Nutr Health Aging. 2010;14(9):782–5.
Krakauer NY, Krakauer JC. A new body shape index predicts mortality hazard independently of body mass index. PLoS One. 2012;7(7):e39504.
Stefanescu A, Revilla L, Lopez T, Sanchez SE, Williams MA, Gelaye B. Using a body shape index (ABSI) and body roundness index (BRI) to predict risk of metabolic syndrome in Peruvian adults. J Int Med Res. 2020;48(1):0300060519848854.
Haghighatdoost F, Sarrafzadegan N, Mohammadifard N, Asgary S, Boshtam M, Azadbakht L. Assessing body shape index as a risk predictor for cardiovascular diseases and metabolic syndrome among Iranian adults. Nutrition. 2014;30(6):636–44.
Rico-Martín S, Calderón-García JF, Sánchez-Rey P, Franco-Antonio C, Martínez Alvarez M, Sánchez Muñoz-Torrero JF. Effectiveness of body roundness index in predicting metabolic syndrome: a systematic review and meta-analysis. Obes Rev. 2020;21(7):e13023.
Taverna MJ, Martínez-Larrad MT, Frechtel GD, Serrano-Ríos M. Lipid accumulation product: a powerful marker of metabolic syndrome in healthy population. Eur J Endocrinol. 2011;164(4):559.
Chiang J-K, Koo M. Lipid accumulation product: a simple and accurate index for predicting metabolic syndrome in Taiwanese people aged 50 and over. BMC Cardiovasc Disord. 2012;12(1):1–6.
Amato MC, Giordano C, Galia M, Criscimanna A, Vitabile S, Midiri M, et al. Visceral adiposity index: a reliable indicator of visceral fat function associated with cardiometabolic risk. Diabetes Care. 2010;33(4):920–2.
Bennasar-Veny M, Lopez-Gonzalez AA, Tauler P, Cespedes ML, Vicente-Herrero T, Yañez A, et al. Body adiposity index and cardiovascular health risk factors in Caucasians: a comparison with the body mass index and others. PLoS One. 2013;8(5):e63999.
Djibo DA, Araneta MRG, Kritz-Silverstein D, Barrett-Connor E, Wooten W. Body adiposity index as a risk factor for the metabolic syndrome in postmenopausal Caucasian, African American, and Filipina women. Diabetes Metab Syndr. 2015;9(2):108–13.
Motamed N, Razmjou S, Hemmasi G, Maadi M, Zamani F. Lipid accumulation product and metabolic syndrome: a population-based study in northern Iran, Amol. J Endocrinol Investig. 2016;39(4):375–82.
Rotter I, Rył A, Szylińska A, Pawlukowska W, Lubkowska A, Laszczyńska M. Lipid accumulation product (LAP) as an index of metabolic and hormonal disorders in aging men. Exp Clin Endocrinol Diabetes. 2017;125(03):176–82.
Goldani H, Adami FS, Antunes MT, Rosa LH, Fassina P, Grave MTQ, et al. Applicatility of the visceral adiposity index (VAI) in the prediction of the components of the metabolic syndrome in elderly. Nutricion hospitalaria. 2015;32(4):1609–15.
Liu P, Ma F, Lou H, Zhu Y. Body roundness index and body adiposity index: two new anthropometric indices to identify metabolic syndrome among Chinese postmenopausal women. Climacteric. 2016;19(5):433–9.
Heshmat R, Heidari M, Ejtahed HS, Motlagh ME, Mahdavi-Gorab A, Ziaodini H, et al. Validity of a continuous metabolic syndrome score as an index for modeling metabolic syndrome in children and adolescents: the CASPIAN-V study. Diabetol Metab Syndr. 2017;9:89.
Ostovar A, Nabipour I, Larijani B, Heshmat R, Darabi H, Vahdat K, et al. Bushehr elderly health (BEH) Programme, phase I (cardiovascular system). BMJ Open. 2015;5(12):e009597.
Shafiee G, Ostovar A, Heshmat R, Darabi H, Sharifi F, Raeisi A, et al. Bushehr elderly health (BEH) programme: study protocol and design of musculoskeletal system and cognitive function (stage II). BMJ Open. 2017;7(8):e013606.
Aadahl M, Jorgensen T. Validation of a new self-report instrument for measuring physical activity. Med Sci Sports Exerc. 2003;35(7):1196–202.
Ervin RB. Prevalence of metabolic syndrome among adults 20 years of age and over, by sex, age, race and ethnicity, and body mass index: United States, 2003-2006. Natl Health Stat Report. 2009;2009(13):1–7.
Bergman RN, Stefanovski D, Buchanan TA, Sumner AE, Reynolds JC, Sebring NG, et al. A better index of body adiposity. Obesity (Silver Spring). 2011;19(5):1083–9.
Kahn HS. The "lipid accumulation product" performs better than the body mass index for recognizing cardiovascular risk: a population-based comparison. BMC Cardiovasc Disord. 2005;5:26.
Thomas DM, Bredlau C, Bosy-Westphal A, Mueller M, Shen W, Gallagher D, et al. Relationships between body roundness with body fat and visceral adipose tissue emerging from a new geometrical model. Obesity (Silver Spring). 2013;21(11):2264–71.
Fluss R, Faraggi D, Reiser B. Estimation of the Youden index and its associated cutoff point. Biom J. 2005;47(4):458–72.
Wang H, Liu A, Zhao T, Gong X, Pang T, Zhou Y, et al. Comparison of anthropometric indices for predicting the risk of metabolic syndrome and its components in Chinese adults: a prospective, longitudinal study. BMJ Open. 2017;7(9):e016062.
Banik SD, Pacheco-Pantoja E, Lugo R, Gómez-de-Regil L, Aké RC, González RMM, et al. Evaluation of anthropometric indices and lipid parameters to predict metabolic syndrome among adults in Mexico. Diabetes Metab Syndr Obes. 2021;14:691.
Erem C, Hacıhasanoglu A, Deger O, Topbaş M, Hosver I, Ersoz HO, et al. Prevalence of metabolic syndrome and associated risk factors among Turkish adults: Trabzon MetS study. Endoctrine. 2008;33(1):9–20.
Barranco-Ruiz Y, Villa-González E, Venegas-Sanabria LC, Chavarro-Carvajal DA, Cano-Gutiérrez CA, Izquierdo M, et al. Metabolic syndrome and its associated factors in older adults: a secondary analysis of SABE Colombia in 2015. Metab Syndr Relat Disord. 2020;18(8):389–98.
Saad MAN, Cardoso GP, de Andrade MW, Velarde LGC, da Cruz Filho RAJABDC. Prevalence of metabolic syndrome in elderly and agreement among four diagnostic criteria. Arq Bras Cardiol. 2014;102(3):263.
Yan H, Zhang M, Zhang X, Xia Y, Shen T, Zhao Z, et al. Study of epidemiological characteristics of metabolic syndrome and influencing factors in elderly people in China. Zhonghua Liu Xing Bing Xue Za Zhi. 2019;40(3):284–9.
Tabatabaei-Malazy O, Saeedi Moghaddam S, Rezaei N, Sheidaei A, Hajipour MJ, Mahmoudi N, et al. A nationwide study of metabolic syndrome prevalence in Iran; a comparative analysis of six definitions. PLoS One. 2021;16(3):e0241926.
Kozan O, Oguz A, Abaci A, Erol C, Ongen Z, Temizhan A, et al. Prevalence of the metabolic syndrome among Turkish adults. Eur J Clin Nutr. 2007;61(4):548–53.
Hosseinpanah F, Asghari G, Barzin M, Golkashani HA, Azizi F. Prognostic impact of different definitions of metabolic syndrome in predicting cardiovascular events in a cohort of non-diabetic Tehranian adults. Int J Cardiol. 2013;168(1):369–74.
Azizi F, Hadaegh F, Khalili D, Esteghamati A, Hosseinpanah F, Delavari A, et al. Appropriate definition of metabolic syndrome among Iranian adults: report of the Iranian National Committee of Obesity. Arch Iran Med. 2010;13(5):426–8.
Bao Y, Lu J, Wang C, Wang C, Yang M, Li H, Zhang X, et al. Optimal waist circumference cutoffs for abdominal obesity in Chinese. Atherosclerosis. 2008;201(2):378–84.
Ritchie S, Connell JJN. The link between abdominal obesity, metabolic syndrome and cardiovascular disease. Nutr Metab Cardiovasc Dis. 2007;17(4):319–26.
Mathieu P, Pibarot P, Larose E, Poirier P, Marette A, Després J-PJTIJOB, et al. Visceral obesity and the heart. Int J Biochem Cell Biol. 2008;40(5):821–36.
Pouliot M-C, Després J-P, Lemieux S, Moorjani S, Bouchard C, Tremblay A, et al. Waist circumference and abdominal sagittal diameter: best simple anthropometric indexes of abdominal visceral adipose tissue accumulation and related cardiovascular risk in men and women. Am J Cardiol. 1994;73(7):460–8.
Motamed N, Khonsari M, Rabiee B, Ajdarkosh H, Hemasi G, Sohrabi M, et al. Discriminatory ability of visceral adiposity index (VAI) in diagnosis of metabolic syndrome: a population based study. Exp Clin Endocrinol Diabetes. 2017;125(03):202–7.
Knowles K, Paiva L, Sanchez S, Revilla L, Lopez T, Yasuda M, et al. Waist circumference, body mass index, and other measures of adiposity in predicting cardiovascular disease risk factors among Peruvian adults. Int J Hypertens. 2011;2011:931402.
Hosseinpanah F, Barzin M, Mirbolouk M, Abtahi H, Cheraghi L, Azizi F. Lipid accumulation product and incident cardiovascular events in a normal weight population: Tehran lipid and glucose study. Eur J Prev Cardiol. 2016;23(2):187–93.
Biyik Z, Guney IJERMPS. Lipid accumulation product and visceral adiposity index: two new indices to predict metabolic syndrome in chronic kidney disease. Eur Rev Med Pharmacol Sci. 2019;23(5):2167–73.
Shin K-A, Kim YJJD. Usefulness of surrogate markers of body fat distribution for predicting metabolic syndrome in middle-aged and older korean populations. Diabetes Metab Syndr Obes. 2019;12:2251.
Wakabayashi I, Daimon TJCCA. The “cardiometabolic index” as a new marker determined by adiposity and blood lipids for discrimination of diabetes mellitus. Clin Chim Acta. 2015;438:274–8.
Zhou C, Zhan L, Yuan J, Tong X, Peng Y, Zha Y, et al. Comparison of visceral, general and central obesity indices in the prediction of metabolic syndrome in maintenance hemodialysis patients. Eat Weight Disord. 2020;25(3):727–34.
Kahn HS. The “lipid accumulation product” performs better than the body mass index for recognizing cardiovascular risk: a population-based comparison. BMC Cardiovasc Disord. 2005;5:26.
Acknowledgments
We would like to thank all the personnel of the Bushehr Elderly Health program and all the individuals who took part in the study.
Author information
Authors and Affiliations
Contributions
All authors read and approved the final paper.
Corresponding authors
Ethics declarations
Conflict of interest
The authors declare that they have no conflict interests.
Additional information
Publisher’s note
Springer Nature remains neutral with regard to jurisdictional claims in published maps and institutional affiliations.
Rights and permissions
About this article
Cite this article
Rabiei, N., Heshmat, R., Gharibzadeh, S. et al. Comparison of anthro-metabolic indicators for predicting the risk of metabolic syndrome in the elderly population: Bushehr Elderly Health (BEH) program. J Diabetes Metab Disord 20, 1439–1447 (2021). https://doi.org/10.1007/s40200-021-00882-4
Received:
Accepted:
Published:
Issue Date:
DOI: https://doi.org/10.1007/s40200-021-00882-4